10 Computer Vision Use Cases in Healthcare
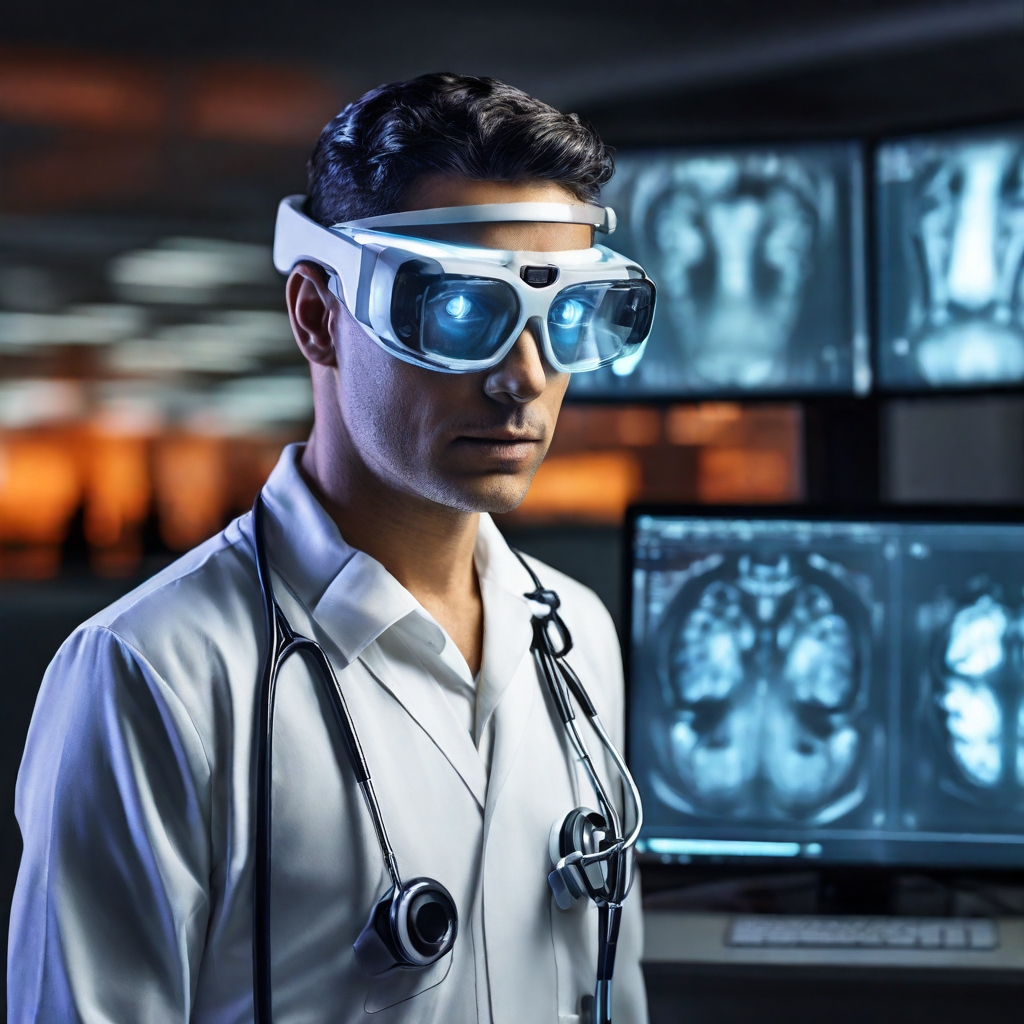
For many governments, the provision of quality healthcare services remains top of mind. In 2020 alone, a total of $13.9billion was spent on digital health funding, and this number is expected to rise in the coming years. These funds are invested in emerging technologies such as artificial intelligence, augmented reality, deep learning, and vision technology.
At present, computer vision is one of the most groundbreaking in the healthcare industry. This field of AI trains computers to see, identify, and process images like humans. This means computers can now not only observe but also make decisions.
The implementation of computer vision in healthcare comes with tremendous benefits. And as a result, many healthcare institutions are jumping on this trend. At Appvales, we have already helped several healthcare organizations develop software that can be used in the application of computer vision in medical field. Our experts have vast experience in building complex software, thus, you can rest assured your project will be in good hands.
Back to the brass tacks. In this insightful article, we are going to explore real-world applications of computer vision in healthcare. Read on to stay informed and ahead of the competition.
Computer Vision Statistics Worth Taking Note of
Computer vision solutions are an aspect of AI that has the potential to be a booming market and opportunity hub in the not-so-distant future. Below are statistics that strongly confirm this:
- The global computer vision market was valued at USD 11.32 billion in 2020 and is expected to expand at a compound annual growth rate (CAGR) of 7.3% from 2021 to 2028. (Grand View Research)
- Clinical health AI applications can potentially create $150 billion in annual savings for the US healthcare economy by 2026.
- In terms of growth, the AI Software Platforms category of the AI market is forecast to be the strongest, with a five-year CAGR of 32.7%. Computer vision solutions fall within this category.
- By 2022, the global market for computer vision is projected to reach $48.6 billion annually, up from just $6.6 billion in 2015.
These numbers clearly show that this is the best time for healthcare providers and other related industries to consider computer vision solutions strongly.
Benefits of Computer Vision for Healthcare
So, what are some of the benefits health centers can expect once they adopt computer vision?
- More accurate diagnosis:
To err is human.’ This is a common saying, which holds truth. However, in a field where diagnoses are a matter of life and death (literally!), it isn’t worth taking the risk: at least if you can avoid it.
The number of unnecessary deaths in the United States is 22,000 lives per year, of which a significant percentage results from inaccurate diagnosis.
Thankfully, applying computer vision in healthcare applications goes a long way in reducing this margin of error. The more data the software receives for algorithm training, the higher its level of accuracy. Considering that 90% of healthcare data comes in the form of images, finding data for your CV software would not be a problem.
- Early disease recognition:
With conditions like cancer and COVID, late detection is one factor that would gravely affect the recovery chances of patients.
However, machine vision medical applications are slowly making these situations something of the past. Conditions like cancer, pneumonia, osteoporosis, etc., can easily be detected early in patients by computer vision, and they would be given treatment quickly.
- Speed and efficiency:
Sometimes, the problem is not that the disease isn’t detected on time. Instead, it could be that it isn’t treated fast or handled effectively enough.
However, speed and efficiency are not a problem when using computer vision in medicine. Also, considering that the United States is currently undergoing a physician shortage during a pandemic, fast and efficient healthcare is needed now more than ever.
- Better medical imaging analysis:
Computer vision allows 3D visualization, which significantly helps in the diagnosis and treatment of certain diseases. 3D images are more informative than the standard 2D, and they help healthcare providers to make more informed decisions concerning patient care.
- Cost reduction:
All the benefits above would eventually lead to a decrease in the cost of healthcare services for patients. Automating healthcare services will lead to efficiency and speed, which would decrease the time a patient would spend either in the hospital or taking medications.
You’ll find that this would reduce the amount of money the patient would’ve spent paying for medications or services.
Computer Vision Use Cases in Healthcare
- Cancer Detection:
One primary use of computer vision in medical field is for early cancer detection. Machine vision can differentiate between cancerous cells and non-cancerous cells based on information from thousands of images and biopsy results.
For instance, SkinVision is an app that successfully uses AI and other technologies to detect 95% of skin cancers. There have also been breakthroughs in detecting breast and lung cancer with this technology.

- Automating Lab Tests:
Blood analyzers that integrate machine vision in their application can run multiple patient results. For example, using computer vision, they can analyze blood count, tissue cells e.t.c by going through several images.
- Tumor detection:
Brain tumors are also one of the health conditions that early detection would go a long way to mitigate its dangerous effects on patients.
Computer vision technologies like MASK-R Convolutional Neural Networks (MASK R-CNN) have been developed to detect tumors in patients and ensure they receive early treatment.
- Medical imaging:
Medical computer vision companies also use this technology for medical imaging. This process uses computer vision and object detection to find anomalies in the scans of various vital organs.
Physicians are leveraging apps like Arterys in MRI scans to make the proper diagnoses.
- Patient monitoring:
Healthcare providers can also use computer vision to monitor the rehabilitation of outpatients and newly discharged patients. That way, healthcare providers can track their patient’s progress virtually.
Within the elderly community, fall detection systems that employ computer vision software and pose estimation. These systems aim at reducing the number of times older adults lose their balance. It also is equipped with technology that would quickly call for help when a user does fall.
- Surgical simulation
Some machine vision medical applications are used for training surgeons. This is to give them an experience of surgery beforehand, thus increasing success rates and reducing risks. Certain apps like TouchVision, use CV technology to create realistic surgery simulations. The AI uses 3D imaging to make this simulation more accurate for the surgeon-in-training.
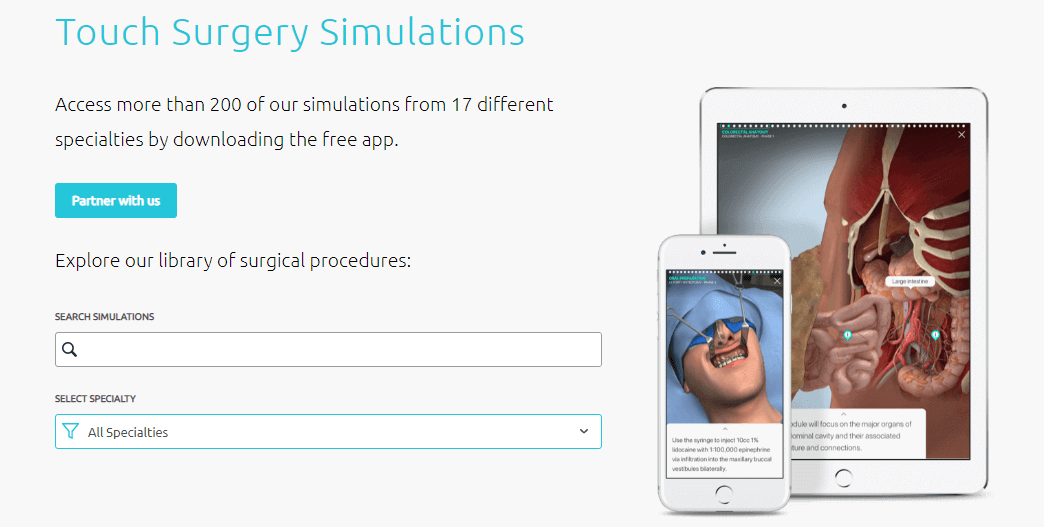
- Pneumonia detection
This pneumonia detection web app is an open-source project that healthcare providers can not yet use for clinical purposes. The app was tested on 500 images of chest x-ray and has achieved 86% accuracy.
Although this use case has not been deployed yet, it shows the potential of computer vision in medicine and diagnostics.
- Accelerated research:
Computer vision has disrupted the world of medical research as well. By analyzing anonymized past patient records, CV-powered applications can detect and identify trends in disease progression. This helps save money and time spent on clinical trials and even discover how to cure the disease entirely.
- Diagnostic use cases:
Microsoft’s InnerEye is a perfect example of image processing applications in healthcare. InnerEye is used to detect tumors and other anomalies in x-rays. By uploading x-ray scans, the program sees and measures aberrations that would otherwise go unnoticed.
- Anti Covid Measures Analysis:
Computer vision has also been of help during this pandemic for COVID diagnosis, prevention, and treatment. Technologies like Masked Face Recognition, computed tomography, germ screening, etc., are ways computer vision has been used to manage COVID. Analysis of chest X-rays has also helped in COVID detection, with some algorithms reaching an accuracy of 97%.
Just recently, Appvales built software that uses computer vision to ascertain if health workers are doing all they can to prevent exposure and transmission of COVID. This app would prove helpful in a hospital setting and would significantly reduce negligence cases on the part of health workers.
Challenges encountered while using Computer Vision in Healthcare
Despite its numerous benefits, computer vision in medicine has not been generally accepted. Many people are skeptical about the use of this AI technology in healthcare for a good number of reasons. Listed below are some of them:
- The consequences of false negatives or false positives:
When used in other industries, errors in computer vision can be simple and, therefore, easy to overlook. But with healthcare, it is a whole different ball game. Errors in diagnosis can lead to health complications and fatal consequences.
And unlike with human error, it is hard or even impossible to pin the blame on software. This can lead to complicated legal issues among healthcare providers, software companies, and the affected patients.
Also, when a technology like computer vision is built with a dataset with an underlying fault, it can injure thousands of patients. This is because multiple units of the technology are most likely being used in different hospitals and healthcare centers. And if they all depend on the faulty dataset in question, then there’s a problem.
But how do software companies fix this and ensure that computer vision can be safe for use?
They stress the need for professional oversight and image labeling by experts. We will go in-depth into image labeling later in this article. For now, let’s consider professional supervision.
Currently, computer vision has not developed to the point of being stand-alone diagnostic equipment. As much as it makes deductions based on statistics and already available data, healthcare providers are still responsible for making the final decision for patients. Computer vision simply enhances their accuracy and makes diagnosis faster.
- Lack of generalization:
Unlike other sectors where computer vision is used, the healthcare sector does not allow imaging generalization.
To explain better, let’s consider when computer vision is used in the automobile industry. It is easy for computer vision to observe and analyze cars because they all have a general shape and structure.
However, in healthcare, organs of different individuals usually have unique structures and sizes. Thus, building a system that automatically detects anatomical structures will not be easy because the software has to note the possibility of a discrepancy in the general size and configuration of organs.
Thankfully, the solution to this challenge has been developed. With semantic image segmentation, computer vision technologies can accurately classify images pixel-by-pixel.
- Unemployment concerns:
The more computer vision develops, the more people are concerned about AI taking over jobs. And sincerely, this concern is too far-fetched.
Humans program computer vision to assist humans. Not to entirely thrust the bulk of the job into digital hands. Also, healthcare is not an industry that can afford the luxury of placing people’s lives completely in the care of artificial technology.
- Professional realignment:
Another concern surrounding the application of computer vision in healthcare affects the medical practitioners involved. The increased use of machine vision in medicine would require a shift in the profession as most of their work will become automatable.
Scholars are concerned that human capacity and knowledge might reduce because of this broad use of computer vision. This would make it harder for healthcare providers to detect any errors that might occur during its use accurately.
A simple solution to this would be healthcare provider training, engagement, and education. Digitization can not be paused for any one sector, so it is in the place of the people involved to prepare themselves for this shift and adjust accordingly.
Medical Image Datasets: How to Acquire them for your Computer Vision Solution
Computer vision technologies depend on huge datasets to be able to function effectively. But enterprises and healthcare industries might have a difficult time finding a suitable dataset.
Before we delve deeper into the methods of dataset acquisition, here are the attributes of a good dataset for computer vision:
- Quality: Images contained in a healthcare dataset must be of high resolution. This would help train the machine vision software and cause it to detect and analyze any anomalies accurately.
- Quantity: While training computer vision technologies, it is impossible to have excess data. The more data there is, the more accurate and intuitive your technology will be.
- Variance: The medical images must vary in age, gender, race, geography, e.t.c. This would make the computer vision technology more effective as it can be used on various patients from different demographics.
- Density: It would help to have images with multiple objects to detect. For instance, mammograms of patients with multiple tumors, etc. This would help reflect real-world health conditions that your computer vision technology might encounter.
When considering acquiring a dataset for your computer vision, there are three general ways to obtain your software data.
- Using a public dataset:
These are also called open-source datasets. They are typically found online and readily available to be used. Some are meant to be purchased, while others are free online.
They’re usually not alterable and they come in different formats like .csv, .json., e.t.c. Building datasets from the ground up usually involve rigorous regulations and costs that startups and smaller enterprises cannot handle. Therefore, open datasets are usually the best option in such cases.
The disadvantage is that this dataset’s features, quality, and pre-labeling might not be a perfect match for your desired computer vision software. Thus, it is good to use public datasets for testing your computer vision concept, but not very advisable for deploying and maintaining computer vision software.
Also, public datasets for computer vision in medical field are not as plentiful as public datasets for other machine learning functions.
However, the chances are that, with an extensive search, you will find one that will suit your needs. Examples of public datasets in healthcare are Chest X-ray Masks and Labels Dataset, NIH Chest X-rays Dataset, Lung Cancer Dataset, e.t.c. Alternatively, Kaggle is a platform that contains over 50,000 datasets. You can source the dataset for your computer vision technology from the vast options in Kaggle.
- Creating a custom dataset for your computer vision software:
You can build your custom dataset from the ground up by yourself or with the services you hire. But before you can develop and use a custom dataset for your technology, there are particular domain-specific challenges you’ll have to overcome. They are:
- Getting ethical approval to obtain medical images:
All medical images are stored in a format known as DICOM (Digital Imaging and Communications in Medicine) DICOM is used as the standard so interoperability can exist between different providers in the healthcare industry.
While developing a dataset for your computer vision software, you need to get approval from the local ethical committee. This is because the DICOM images contain the personal health information (PHI) of patients, and this information is protected by the Health Information Portability and Accountability Act (HIPAA) and the General Data Protection Regulation (GDPR).
Using DICOM files without adequate authorization can lead to dire consequences for the parties involved. This is why seeking permission is necessary.
- Anonymizing the data to comply with privacy regulations:
When the ethical committee approves your request, the next step is to anonymize the DICOM files to protect the PHI of the patients. This must be done before the data is fed to the computer vision algorithm.
Anonymizing simply means erasing and losing all forms of PHI associated with the DICOM files. Specific tools, such as DICOM Anonymizer, automatically erase PHI from medical image files and replace the information with dummy values.
- Labeling data to create a ground-truth dataset:
After anonymizing, the next step is image labeling or annotation. This step requires engaging radiologists to go over each image in the dataset thoroughly. They do this to develop a ground-truth dataset, the final dataset that your computer vision technology will be deployed with.
This step is very time-consuming and expensive. Also, to comply with the FDA and their rule concerning machine learning-based technology in healthcare, the image dataset must indicate the experts who annotate it.
There are two types of image annotations:
- Semantic segregation- In this type, the radiologists mark an area of interest in the image file. It might be an organ, malignant cell, lesion, abnormality, e.t.c. This type of labeling helps when using computer vision for object detection or to identify anatomical structures.
- Classification- Here, the images are categorized based on the absence or presence of a particular disease.
Both types are used simultaneously while building a ground-truth dataset. This is so the computer vision technology can detect a disease, e.g., a tumor, in an image and differentiate it from other cells.
After the ground-truth dataset has been developed, you and your software team can use it to program your computer vision technology.
- Partnering with a Third-party to create a Custom Dataset:
Here, you can partner with an external organization or vendor to do the data collecting for you. This vendor will handle everything surrounding the building of the dataset. This includes the legal and ethical requirements necessary.
This option is ideal when you do not have the resources to create the custom dataset for your machine vision software. With a third-party vendor, you will still be able to oversee the building of the dataset and ensure that it is according to your desired specifications.
It is also an excellent way to leverage the vendor’s expertise in creating datasets for different use cases to ensure that your technology is programmed with the most suitable custom dataset.
The disadvantage to this option is that the cost of hiring a third party vendor might be high.
In Conclusion
As much as there is still much to be achieved, computer vision is not just a trend. It is an efficient and long-term solution in healthcare. It shows great potential in the provision of quality and accurate services. Hence, to ensure efficiency, programmers continue to develop it to ensure maximum efficiency.If you are looking to build healthcare software, Appvales has got you covered. We will conceive your ideas, brainstorm, and develop software best suited for your facility. With a pool of talented healthcare software developers, you are assured of quality, efficient, and effective applications. Contact us today.